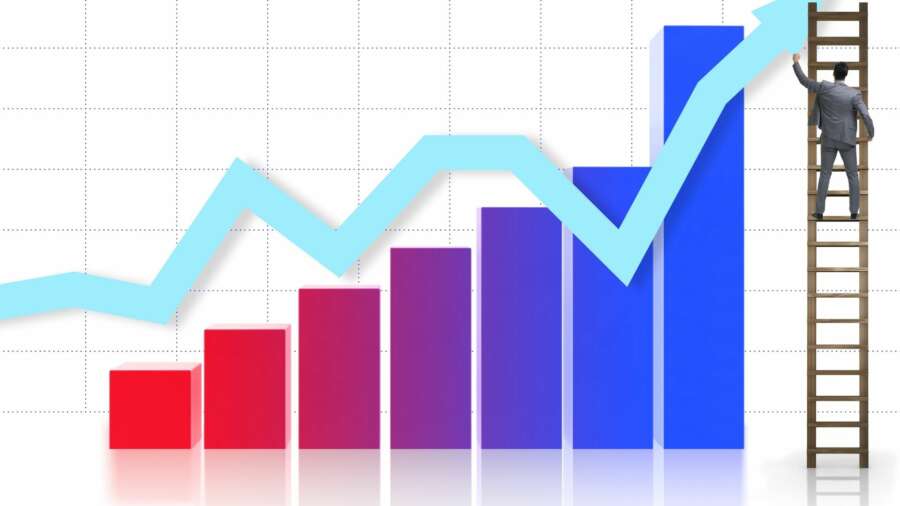
There is little difference between a parameter vs statistic. They are two terms that describe the same thing. But often they are used interchangeably. This can cause confusion, and you may not know which one to use. If you are confused about what to use, then here are some guidelines to help you make the decision.
PARAMETER – A parameter refers to an estimator or a rule that is used in making predictions about a particular variable. The best example of a parameter is the speed of light. The speed of light can be measured in many different ways, but it is estimated by scientists through a mathematical equation that describes the speed of light.
PARAMETER – A parameter refers to a rule or an estimator that is used in making predictions about a particular variable. The best example of a parameter is the speed of light.
STATISTICS – The term statistic refers to the process of determining how something changes after a measurement has been taken. For example, if a person takes a walk, he or she will measure how far the person walks. Once this information is recorded, a statistician will use the data to predict how long the walk will last. So, instead of taking a walk and measuring how far the person walked, a statistician will take a walk and calculate how long the person walked and then predict how long the walk will last based on the information.
The difference between the two types of statistics is that in a parameter it is dependent upon the measurement, whereas in statistics it is independent. This means that the data will change as the measurements are made, which is how a statistician uses the data to predict how long the process of measurement will last.
In addition to these differences there are a couple of other reasons why a parameter refers to a statistic. One reason is that it is possible to use the same data to make predictions about many different variables. However, there is only a limited number of variables in a set, which makes it difficult to use a single measurement to predict how each variable will behave.
Another reason is that many different types of parameter and statistic can be compared at the same time. If a single value is used, then it can be compared to many different values to see which ones provide a reliable estimate of the value.
There are some things to remember when comparing these types of statistics. For example, a parameter refers to an estimator, while a statistic refers to an actual measurement. In addition, a parameter refers to something that depends on something else, which makes it difficult to make a prediction. So, when comparing them, make sure you get both types of information in order to make an accurate comparison.
When it comes to choosing a statistical model or algorithm, make sure that it includes a lot of parameters. This is because the model will use the numbers to predict the likelihood that a model can make the right prediction.
Statistical models are usually much more accurate than average. When a model can correctly predict the outcome of a measurement, it is able to accurately provide an idea about what the effect would be if the measurement was made.
Many people have said that a parameter is a more useful way of determining how long something will last. If we think about the fact that the measurement is random, it makes it less likely that a model will accurately predict the value. This is because it is harder to predict the value when the measurement is random.
However, the difference between the two types of statistics is not that big of a deal, if you look at it more closely. In most cases the parameter is much more reliable than a statistic, because the data is already known.